Resumes
Resumes
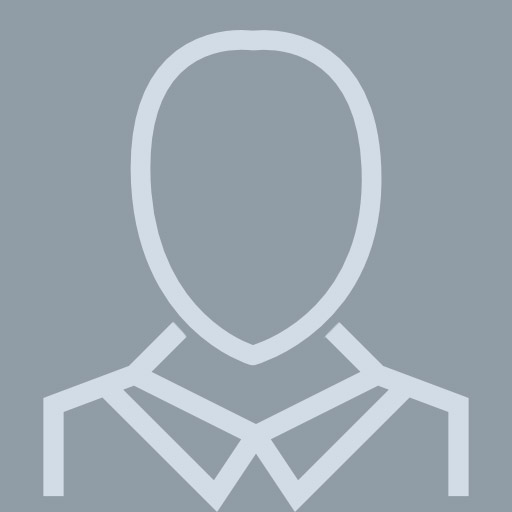
Phd Candidate
View pageLocation:
Pittsburgh, PA
Industry:
Higher Education
Work:
Carnegie Mellon University since Jul 2004
PhD Candidate
Intel May 2007 - Aug 2007
Graduate Student Intern at Strategic CAD Lab
Intel Jun 2003 - Jun 2004
Graduate Student Intern at Microprocessor Research Lab
Oregon State University Sep 2001 - Jun 2003
Graduate Research/Teaching Assistant
Mentor Graphics Jun 2001 - Sep 2001
High Level Synthesis Intern
PhD Candidate
Intel May 2007 - Aug 2007
Graduate Student Intern at Strategic CAD Lab
Intel Jun 2003 - Jun 2004
Graduate Student Intern at Microprocessor Research Lab
Oregon State University Sep 2001 - Jun 2003
Graduate Research/Teaching Assistant
Mentor Graphics Jun 2001 - Sep 2001
High Level Synthesis Intern
Education:
Carnegie Mellon University 2004 - 2010
PhD, Electrical and Computer Engineering Oregon State University 2003 - 2004
MBA Oregon State University 2002 - 2004
MS, Electrical and Computer Engineering Oregon State University 1997 - 2003
BA, International Studies Oregon State University 1997 - 2002
BS, Computer Science Oregon State University 1997 - 2001
BS, Electrical and Electronic Engineering Oregon State University 1997 - 2001
BS, Computer Engineering
PhD, Electrical and Computer Engineering Oregon State University 2003 - 2004
MBA Oregon State University 2002 - 2004
MS, Electrical and Computer Engineering Oregon State University 1997 - 2003
BA, International Studies Oregon State University 1997 - 2002
BS, Computer Science Oregon State University 1997 - 2001
BS, Electrical and Electronic Engineering Oregon State University 1997 - 2001
BS, Computer Engineering
Skills:
Computer Hardware