Resumes
Resumes
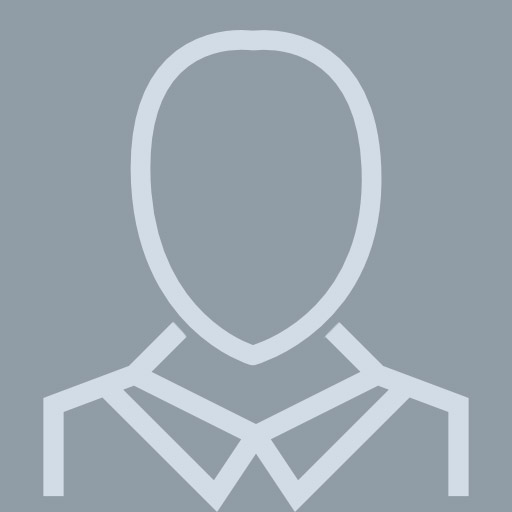
Machine Learning Engineer
View pageLocation:
San Francisco, CA
Industry:
Biotechnology
Work:
Google Apr 2019 - Nov 2016
Software Engineer
Freenome Apr 2019 - Nov 2016
Machine Learning Engineer
Silicon Valley Ai Lab Nov 2016 - Dec 2017
Software Engineer
Microsoft Jun 2014 - Sep 2014
Software Development Engineer Intern
Mit Little Devices Lab Jan 2014 - Mar 2014
Software Development Intern
Software Engineer
Freenome Apr 2019 - Nov 2016
Machine Learning Engineer
Silicon Valley Ai Lab Nov 2016 - Dec 2017
Software Engineer
Microsoft Jun 2014 - Sep 2014
Software Development Engineer Intern
Mit Little Devices Lab Jan 2014 - Mar 2014
Software Development Intern
Education:
University of Oxford 2015
Bachelors, Bachelor of Arts Dartmouth College 2011 - 2015
Bachelors, Bachelor of Arts, Mathematics, Computer Science, Economics University of Oxford 2014 - 2014
Montgomery Blair High School 2007 - 2011
Bachelors, Bachelor of Arts Dartmouth College 2011 - 2015
Bachelors, Bachelor of Arts, Mathematics, Computer Science, Economics University of Oxford 2014 - 2014
Montgomery Blair High School 2007 - 2011
Skills:
Tensorflow
Cuda
Python
C
C++
Java
Numpy
Scipy
Machine Learning
Statistics
Algorithms
Data Analysis
Probability
Parallel Computing
Software Development
Cuda
Python
C
C++
Java
Numpy
Scipy
Machine Learning
Statistics
Algorithms
Data Analysis
Probability
Parallel Computing
Software Development